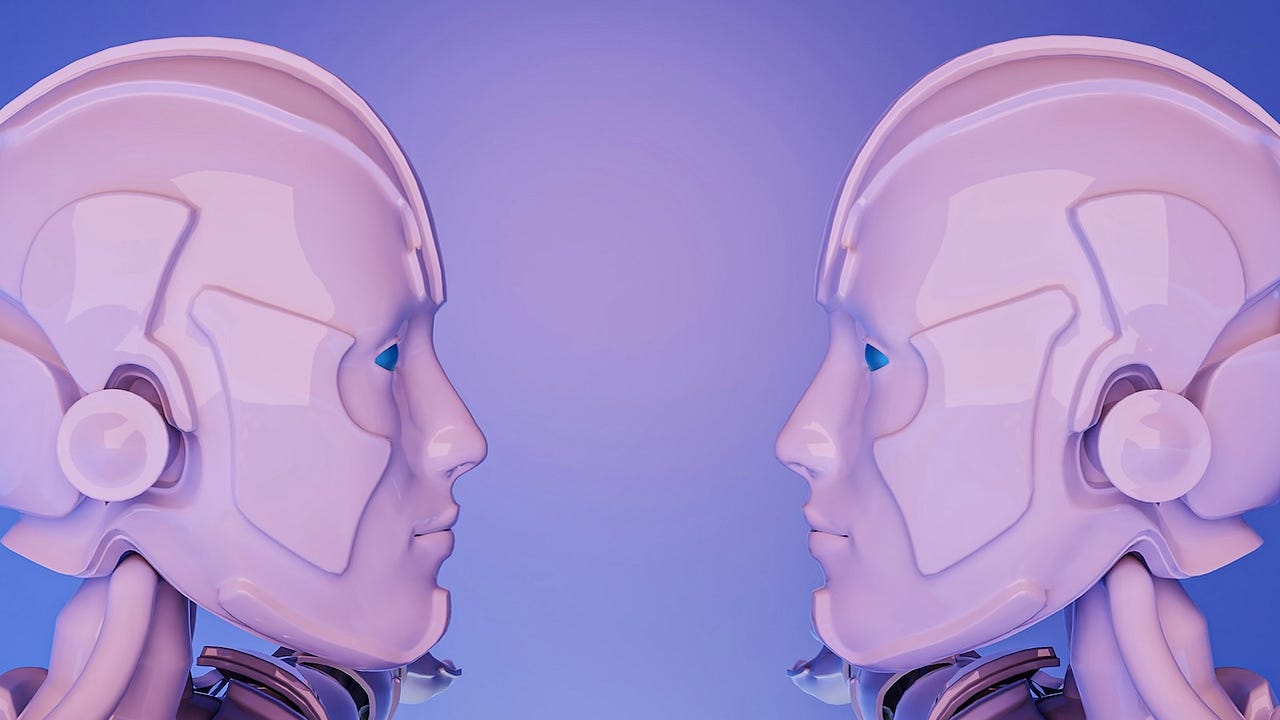
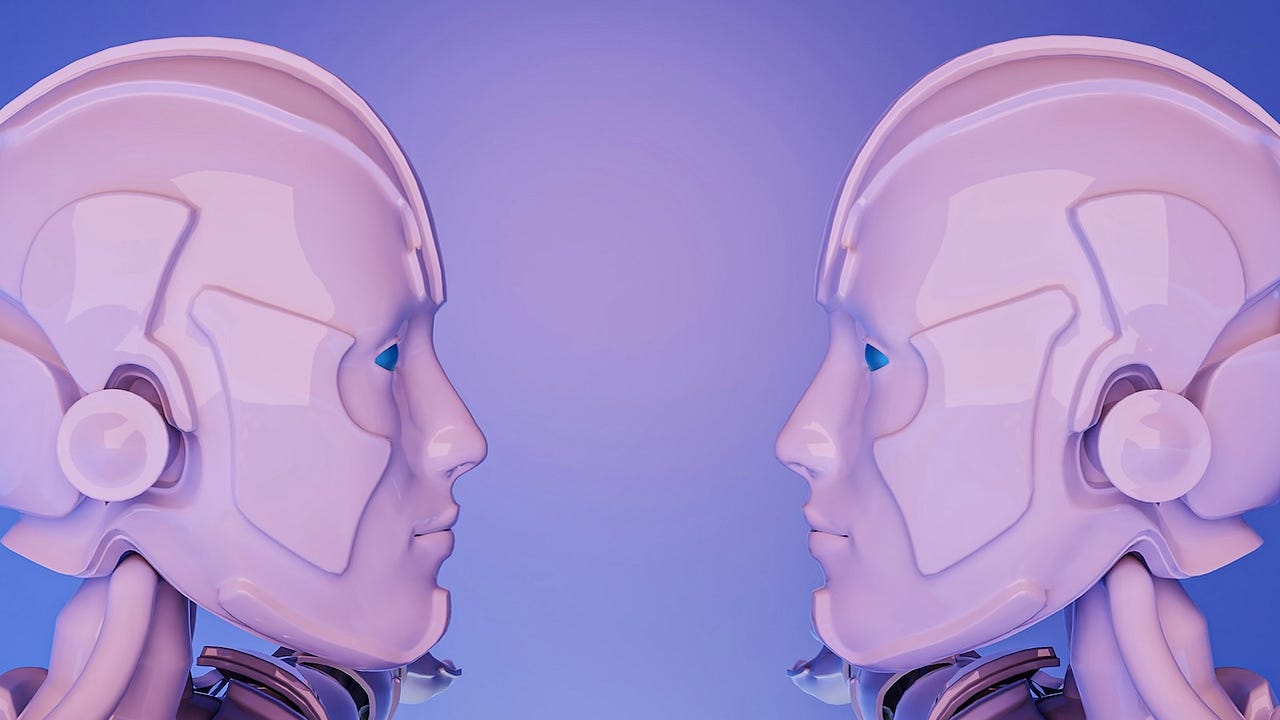
Watching AI Talk to AI is Fascinating, Scary and Observable
AI-to-AI conversations, decoded and on display. No black boxes. No guesswork.
READ THE LATEST
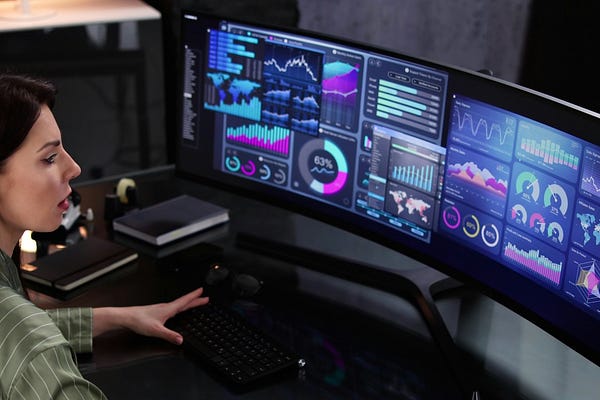
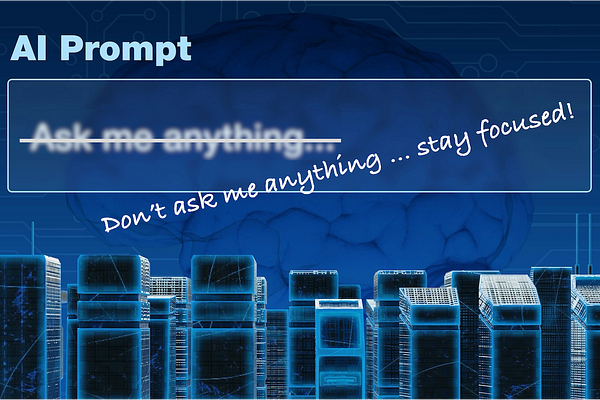
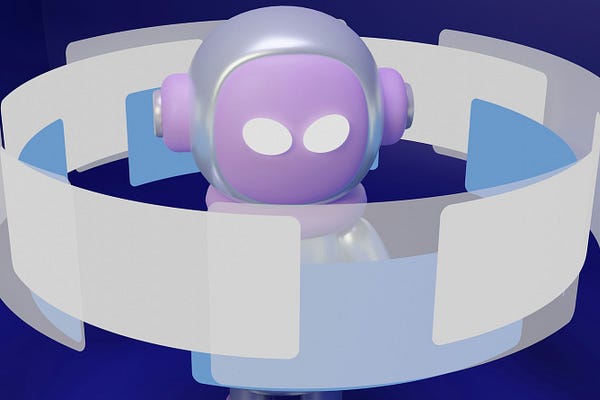

Inside Charli AI Labs
An exclusive look behind the scenes at Charli AI Labs and the cutting-edge advancements in Multidimensional AI™ for finance and capital markets. From deep research to real-time what-if analysis, we show how our AI cuts through the noise.
© 2025 Kevin Collins
Substack is the home for great culture